Behind the Scenes of Algorithmic Recommendations
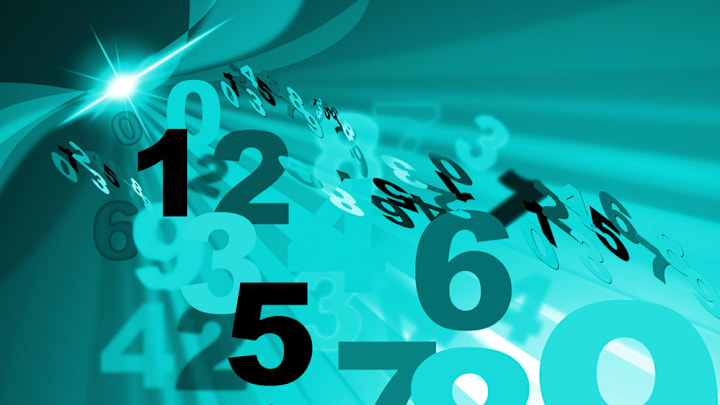
Ever wondered how Netflix suggests that eerily perfect series right after you finish a show? Or how your music app lines up a playlist that somehow matches your exact vibe? Or why your social feed is filled with oddly specific content that’s... a little too relevant?
That’s not coincidence—it’s the quiet handiwork of algorithmic recommendation systems, humming along in the background of nearly every digital experience. These invisible curators sift through mountains of data to guess what you’ll like, want, or need next. And whether you're shopping, scrolling, or streaming, they’re shaping your choices more than you probably realize.
But how do they actually work? And what are we giving up in exchange for all this personalized convenience?
Let’s lift the digital curtain and take a closer look at how algorithmic recommendations work—and how they’re subtly reshaping our online lives.
What Is a Recommendation Algorithm, Anyway?
At its simplest, a recommendation algorithm is a system that predicts what content or products you’ll like based on patterns in your behavior—and often, the behavior of others.
These systems are used across platforms to serve up:
- Movies or shows on streaming services
- Products in online stores
- Videos on platforms like YouTube or TikTok
- Songs on music apps
- Posts and people in social media feeds
They help you discover things faster—but they also help platforms keep you engaged longer. And that’s where the story starts to get interesting.
The Building Blocks of Recommendation Engines
There are a few key strategies most algorithms rely on:
1. Collaborative Filtering
This method says: people like you also liked this. It looks at your past behavior (likes, watches, purchases) and compares it with similar users. If others with your taste loved a certain movie, chances are, you will too.
2. Content-Based Filtering
Here, the system focuses on the attributes of what you’ve liked—genre, artist, price, category—and recommends similar things. Like a moody indie drama? Here's another with the same tone and themes.
3. Hybrid Models
Most modern platforms combine both techniques (and more) into a hybrid system. They also incorporate machine learning to improve over time as they collect more data.
And yes, they’re constantly learning from:
- Your clicks
- Your scroll time
- Your shares and saves
- Your purchases
- Even what you don’t click
Every move you make is a clue.
Why Platforms Love Recommendations
Algorithmic recommendations do more than make life easier for users—they drive engagement and revenue.
- Netflix estimates that over 80% of what people watch comes from its recommendations.
- Amazon owes a significant chunk of its sales to its product suggestion engine.
- TikTok’s For You Page is so fine-tuned that it turns unknown creators into viral stars overnight.
By predicting your interests, platforms increase time spent on site, boost ad impressions, and improve customer satisfaction—all without needing human curation.
The Double-Edged Sword of Personalization
While recommendation algorithms feel helpful, they also raise some important concerns.
1. Filter Bubbles
When you’re only shown what you’re predicted to like, your worldview can narrow. This is particularly dangerous with news and information, where personalization can lead to ideological echo chambers.
2. Manipulation and Profit Bias
Not all recommendations are neutral. Some platforms prioritize content or products that generate more revenue—even if they’re not the best fit for you.
3. Discovery Dilemma
By focusing so much on “what’s likely,” algorithms can stifle serendipity. You’re less likely to stumble upon something totally new, different, or challenging if the system thinks you’ll skip it.
4. Privacy Trade-Offs
To work well, recommendation engines need data—lots of it. This raises ongoing questions about consent, surveillance, and how our preferences are tracked, stored, and monetized.
Can We Outsmart the Algorithm?
Sort of.
While we can’t see or tweak most recommendation engines directly, we can take steps to reclaim a bit of agency:
- Actively seek variety: Watch, listen to, or buy things outside your typical pattern to shake up your profile.
- Use private or incognito mode when you don’t want actions influencing recommendations.
- Adjust your settings: Some platforms let you reset or fine-tune your interests.
- Be mindful of engagement: A long pause or like sends a strong signal—even if it was accidental.
Algorithms are powerful—but they’re still reactive. You’re not trapped, but it does take effort to avoid drifting into autopilot.
The Future: More Personalized, More Invisible
Recommendation systems are only getting smarter. Advances in AI are making them more context-aware, emotion-sensitive, and adaptive. We’ll soon see systems that respond not just to what we do, but how we feel, what we say, and the way we interact with content in real time.
This opens exciting possibilities—especially in education, health, and productivity. But it also demands more transparency, ethical oversight, and user control.
Because while it’s amazing that a platform can finish your thought, the real question is: who’s shaping that thought to begin with?
Curated Convenience or Algorithmic Autopilot?
Algorithmic recommendations are one of the quietest forces guiding our digital lives. They help us find, learn, and enjoy more than we ever could on our own. But they also nudge, filter, and shape our experiences—often without us noticing.
So the next time something “just shows up” in your feed and feels oddly perfect, remember: it probably didn’t land there by chance. It was chosen for you.
And in a world where algorithms make so many decisions, staying curious—and critical—might just be the most important recommendation of all.